Results Cohen's D Article
The effect size for this analysis (d = 1.56) was found to exceed Cohen’s (1988) convention for a large effect (d=.80). These results indicate that individuals in the experimental psychotherapy group (M= 8.45, SD= 3.93) experienced fewer episodes of self- injury following treatment than did individuals in the control group (M= 13.83, SD= 2.14).
- Results: Infection rates ranged from approximately 74 percent to approximately 90 percent, according to levels of psychological stress, and the incidence of clinical colds ranged from approximately 27.
- Even more dismaying is the fact that in seven articles, at least one of the null hypotheses was the research hypotheses, and the nonsignificance of the result was taken as confirmatory; the median power of these tests to detect a medium effect at the two-tailed.05 level was.25! In only two of the articles.
- Cohen’s D is one of the most common ways to measure effect size. An effect size is how large an effect of something is. For example, medication A has a better effect than medication B.
As you read educational research, you’ll encounter t-test (t) and ANOVA (F) statistics frequently. Hopefully, you understand the basics of (statistical) significance testing as related to the null hypothesis and p values, to help you interpret results. If not, see the Significance Testing (t-tests) review for more information. In this class, we’ll consider the difference between statistical significance and practical significance, using a concept called effect size.
The “Significance” Issue
Most statistical measures used in educational research rely on some type of statistical significance measure to authenticate results. Recall that the one thing t-tests, ANOVA, chi square, and even correlations have in common is that interpretation relies on a p value (p = statistical significance). That is why the easy way to interpret significance studies is to look at the direction of the sign (<, =, or >) to understand if the results are statistically meaningful.
While most published statistical reports include information on significance, such measures can cause problems for practical interpretation. For example, a significance test does not tell the size of a difference between two measures (practical significance), nor can it easily be compared across studies. To account for this, the American Psychological Association (APA) recommended all published statistical reports also include effect size (for example, see the APA 5th edition manual section, 1.10: Results section). Further guidance is summed by Neill (2008):
- When there is no interest in generalizing (e.g., we are only interested in the results for the sample), there is no need for significance testing. In these situations, effect sizes are sufficient and suitable.
- When examining effects using small sample sizes, significance testing can be misleading. Contrary to popular opinion, statistical significance is not a direct indicator of size of effect, but rather it is a function of sample size, effect size, and p level.
- When examining effects using large samples, significant testing can be misleading because even small or trivial effects are likely to produce statistically significant results.
What is Effect Size?
The simple definition of effect size is the magnitude, or size, of an effect. Statistical significance (e.g., p < .05) tells us there was a difference between two groups or more based on some treatment or sorting variable. For example, using a t-test, we could evaluate whether the discussion or lecture method is better for teaching reading to 7th graders:
For six weeks, we use the discussion method to teach reading to Class A, while using the lecture method to teach reading to Class B. At the end of the six weeks, both groups take the same test. The discussion group (Class A), averages 92, while the lecture group (Class B) averages 84.
Recalling the Significance Testing review, we would calculate standard deviation and evaluate the results using a t-test. The results give us a value for p, telling us (if p <.05, for example) the discussion method is superior for teaching reading to 7th graders. What this fails to tell us is the magnitude of the difference. In other words, how much more effective was the discussion method? To answer this question, we standardize the difference and compare it to 0.
Effect Size (Cohen’s d, r) & Standard Deviation
Effect size is a standard measure that can be calculated from any number of statistical outputs.
One type of effect size, the standardized mean effect, expresses the mean difference between two groups in standard deviation units. Typically, you’ll see this reported as Cohen’s d, or simply referred to as “d.” Though the values calculated for effect size are generally low, they share the same range as standard deviation (-3.0 to 3.0), so can be quite large. Interpretation depends on the research question. The meaning of effect size varies by context, but the standard interpretation offered by Cohen (1988) is:
.8 = large (8/10 of a standard deviation unit)
.5 = moderate (1/2 of a standard deviation)
.2 = small (1/5 of a standard deviation)

*Recall from the Correlation review r can be interpreted as an effect size using the same guidelines. If you are comparing groups, you don’t need to calculate Cohen’s d. If you are asked for effect size, it is r.
Calculating Effect Size (Cohen’s d)
Option 1 (on your own)
Given mean (m) and standard deviation (sd), you can calculate effect size (d). The formula is:
| m1 (group or treatment 1) – m2 (group or treatment 2) |
[pooled] sd |
Where pooled sd is *√sd1+sd2/2]
Option 2 (using an online calculator)
If you have mean and standard deviation already, or the results from a t-test, you can use an online calculator, such as this one. When using the calculator, be sure to only use Cohen’s d when you are comparing groups. If you are working with correlations, you don’t need d. Report and interpret r.
Wording Results
The basic format for group comparison is to provide: population (N), mean (M) and standard deviation (SD) for both samples, the statistical value (t or F), degrees freedom (df), significance (p), and confidence interval (CI.95). Follow this information with a sentence about effect size (see red, below).
Effect size example 1 (using a t-test): p ≤ .05, or Significant Results
Among 7th graders in Lowndes County Schools taking the CRCT reading exam (N = 336), there was a statistically significant difference between the two teaching teams, team 1 (M = 818.92, SD = 16.11) and team 2 (M = 828.28, SD = 14.09), t(98) = 3.09, p ≤ .05, CI.95 -15.37, -3.35. Therefore, we reject the null hypothesis that there is no difference in reading scores between teaching teams 1 and 2. Further, Cohen’s effect size value (d = .62) suggested a moderate to high practical significance.
Effect size example 2 (using a t-test): p ≥ .05, or Not Significant Results
Among 7th graders in Lowndes County Schools taking the CRCT science exam (N = 336), there was no statistically significant difference between female students (M = 834.00, SD = 32.81) and male students (841.08, SD = 28.76), t(98) = 1.15 p ≥ .05, CI.95 -19.32, 5.16. Therefore, we fail to reject the null hypothesis that there is no difference in science scores between females and males. Further, Cohen’s effect size value (d = .09) suggested low practical significance.
Repeat after me: “statistical significance is not everything.”
It’s just as important to have some measure of how practically significant an effect is, and this is done using what we call an effect size.
Cohen’s d is one of the most common ways we measure the size of an effect.
Here, I’ll show you how to calculate it. If you’d rather skip all that, you can download a free spreadsheet to do the dirty work for you right here. Just use this form to sign up for the spreadsheet, and for more practical updates like this one:
(We will never share your email address with anybody.)
The spreadsheet will also share a confidence interval and margin of error for your Cohen’s d.
The Formula
Cohen’s d is simply a measure of the distance between two means, measured in standard deviations. The formula used to calculate the Cohen’s d looks like this:
Where M1and M2 are the means for the 1st and 2nd samples, and SDpooled is the pooled standard deviation for the samples. SDpooled is properly calculated using this formula:
In practice, though, you don’t necessarily have all this raw data, and you can typically use this much simpler formula:
The spreadsheet I’ve included on this page allows you to use either formula.
In the first, more lengthy formula, X1 represents a sample point from your first sample, and Xbar1 represents the sample mean for the first sample. The distance between the sample mean and the sample point is squared before it is summed over every sample point (otherwise you would just get zero). Obviously, X2 and Xbar2 represent the sample point and sample mean from the second sample. n1 and n2 represent the sample sizes for the 1st and 2nd sample, respectively.
In the second, simpler formula, SD1 and SD2 represent the standard deviations for samples 1 and 2, respectively.
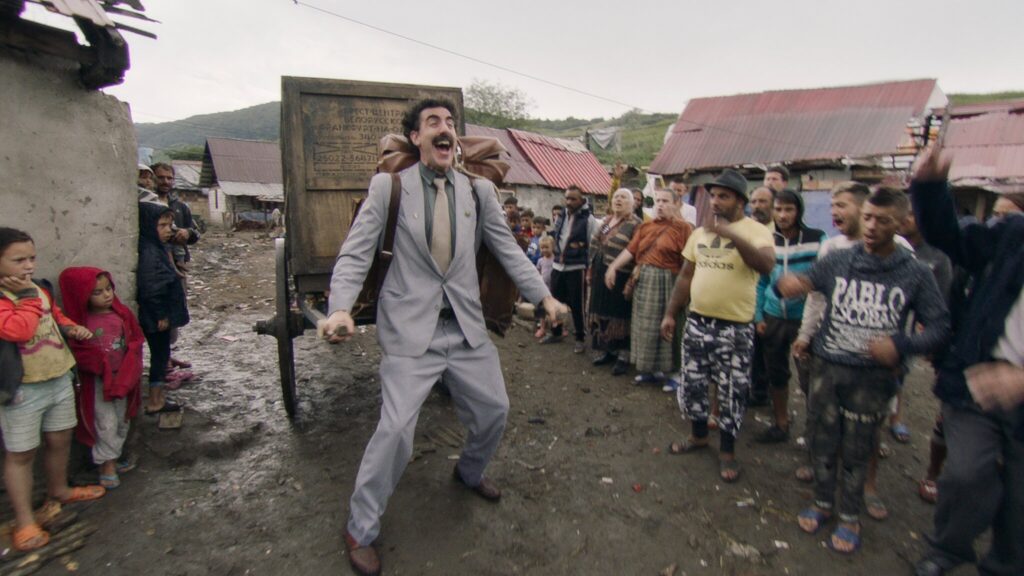
Now for a few frequently asked questions.
Can your Cohen’s d have a negative effect size?
Yes, but it’s important to understand why, and what it means. The sign of your Cohen’s d depends on which sample means you label 1 and 2. If M1is bigger than M2, your effect size will be positive. If the second mean is larger, your effect size will be negative.
In short, the sign of your Cohen’s d effect tells you the direction of the effect. If M1 is your experimental group, and M2 is your control group, then a negative effect size indicates the effect decreases your mean, and a positive effect size indicates that the effect increases your mean.
How is Cohen’s d related to statistical significance?
It isn’t.
It’s important to understand this distinction.
To say that a result is statistically significant is to say that you are confident, to 100 minus alpha percent, that an effect exists. Statistical significance is about how sure you are that an effect is real; it says nothing about the size of the effect.
By contrast, Cohen’s d and other measures of effect size are just that, ways to measure how big the effect is (and in which direction). Cohen’s d tells you how big the effect is compared to the standard deviation of your samples. It says nothing about the statistical significance of the effect. A large Cohen’s d doesn’t necessarily mean that an effect actually exists, because Cohen’s d is just your best estimate of how big the effect is, assuming it does exist.
(Of course, if you have a confidence interval for your Cohen’s d, then the confidence interval can tell you whether or not the effect is significant, depending on whether or not it contains 0.)
Can you convert between Cohen’s d and r, and if so, when?
There is a relationship between Cohen’s d and correlation (r). The following formula is most commonly used to calculate d from r:
And this formula is used to find r from d:
Where a is a correlation factor found using the sample sizes:
However, it’s important to realize that these conversions can sometimes change your interpretation of the data, in particular when base rates are important. You can find an in depth academic discussion of conversions between the two in this paper.
For conversions between d and the log odds ratio, you can also take a look at this paper.
Can you statistically compare two independent Cohen’s d results?
Yes, but not at face value, and only with extreme caution.
Remember, Cohen’s d is the difference between two means, measured in standard deviations. If two experiments are sampled from different populations, the standard deviations are going to be different, so the effect size will also be different.
For example, you can’t compare the effect size of an antidepressant on depressed people with the effect size of an antidepressant on schizophrenic people. The inherent variance of the sample populations are going to be different, so the resulting effect sizes are also going to be different.
Assuming that the experiments were both conducted on the same population, it’s still not a good idea to compare Cohen’s d results at face value. If one value is larger, this doesn’t mean there is a statistically significant difference between the two effect sizes.
The simplest way to compare effect sizes is by their confidence intervals
If the confidence intervals overlap, the difference isn’t statistically significant. To find the confidence interval, you need the variance. The variance of the Cohen’s d statistic is found using:
You can use this variance to find the confidence interval. You can also use the spreadsheet I’ve provided on this page to get the confidence interval.
Can you calculate Cohen’s d from the results of t-tests or F-tests?
Results Cohen's D Articles
Yes, you can. This paper explains how to do that beautifully. If there’s enough demand for it, I might put together a spreadsheet for this also.
Negative Cohen's D
Want to download the Cohen’s d spreadsheet and let it do the dirty work? Sign up here: